Monte Carlo Methods On NISQ devices, Applications for Risk Management
Niklas Hegemann, Co-Founder and Managing Director at JoS QUANTUM
Quantum computing promises improvements in many areas, from chemistry to healthcare to finance. The current investment boost in the field gives hope that quantum computers will become reality in business workflows. Germany alone has pledged 2 billion euros for quantum technologies as part of the Corona measures. This latest article explores what the company JoS is doing in the Quantum space.
Qubit 101
But what is the current state of quantum computing and what news to watch out for to not get lost in all the noise? This article shows the current state of quantum computers along with a potential business example in risk management. Let’s start with the basics. The basic unit in the classical computer is the bit, an object which is either 0 or 1 at a given time. The quantum counterpart is the quantum bit (qubit for short). A qubit is a vector in a two dimensional complex vector space. A quantum computation is a sequence of length preserving operations on such a vector. So one can think of a quantum computation as a product of matrices, acting on a vector. These operations take place in the complex vector space. If we now want to know the result of our operations, we project the results onto a system known to us, e.g. the zeros and ones of the classical computer, this is called measurement. So at the end of our quantum computation we have classical information that we can use e.g. in classical algorithms. An important remark is that quantum algorithms are probabilistic algorithms. This means that we can run the exact same algorithm twice and get different results. Therefore quantum algorithms need to be executed several times to get the desired result with a certain confidence.
Probabilistic Outcomes
When it comes to probabilistic and stochastic processes these properties can be very useful as e.g. drawing perfect random numbers is inherently implemented by nature. Also when we think about probabilities and correlated random variables quantum algorithms offer advantages due to the complex probability amplitudes that can interfere and cancel out that are much more convenient to model probabilities and correlations of complex systems. Therefore alone Monte Carlo methods seem to be a
perfect match for quantum computers.
Everything we described above can be done on a classical computer as well. Classical computers can store and rotate vectors, as well as model the quantum measurement process. So a classical computer can surely simulate a quantum computer to arbitrarily good accuracy. But we have to keep in mind how long such a simulation takes on a classical computer and what resources in terms of memory would be
necessary. Let’s assume we want to simulate 100 qubits. To process this quantum state in the general case we would have to write down 10,000,000,000,000,000,000,000,000,000,000 complex numbers and manipulate these for the computation. No computer that currently exists or is planned can do this in
any foreseeable future. Of course, this realisation has led to the fact that a quantum computer with several qubits, if functional, can make calculations possible that are classically unfeasible. So we are not talking about doing things 10 or 100 times faster but about getting business value from things that used to be impossible before.
A drastic example for this is the application of prime factorization of large numbers – the key ingredient to state-of-the-art encryption methods – which would take billions of years on a classical supercomputer but only minutes or hours on a quantum computer. A further example is the sensitivity analysis of e.g. business risk models
Risk Models
In such risk models , several risk factors with interconnections affect the revenue of the business operations. What is the possible loss for the next year, is there a scenario which might be devastating for the company? A pandemic or regional war can trigger high exchange rate volatility and downgradings of credit ratings, for example. This could subsequently trigger lower growth rates and higher unemployment rates, all with certain probabilities and impacts. Strategically, having good estimates for the likelihood of such events could be very beneficial as companies could identify weak spots in their business model and mitigate certain risks. This would enable Enterprise Risk Management to spot and mitigate risks that are currently invisible, rather than reacting to adverse events after the fact.
Once the inputs have been chosen, this type of calculation takes about 15 minutes on modern infrastructure. If we now change one of the risk parameters and want to see how this change affects the output of our model, we have to wait another 15 minutes.
This leads to the fact that the more input parameters we want to test, the longer the calculation will be. If we change all possible pairs of input parameters, i.e. two per run, it takes 13 years for a model with 1000 input parameters! However, we can represent this model as a quantum circuit and evaluate it by means of a quantum algorithm. Here we find operations on the quantum computer that have a similar or the same output as our classical simulation. If we now set up the same model as a quantum circuit with 200 qubits, the computer would only need 20 minutes of computing time.
What does it mean now? We need 200 of these quantum bits – on which we can perform our quantum operations – to overcome the current technological hurdle.
Unfortunately it is not that simple. Qubits are very error-prone and require a very good quality in order to not lose their state during the calculation. In our example, the operations must be performed so well on the qubits that they succeed without error 99.999999% of the time. Currently commercially available systems have a 99.95% success rate
So the error rate needs to improve by a factor of 10000 before we could successfully run it on a real quantum computer. Of course, this measure is very simplified and the situation is much more complex than described, but it gives a feeling of where quantum computing is right now. So it will be some time before quantum computers have the qubit and operations quality to perform the examples described above.
The natural question is, are there methods to run the algorithms on near term devices with a lower qubit quality? In our recent work published as a preprint on arxiv, we present a new method to solve such problems on a near term quantum computer with a low qubit quality and operation quality. Our approach parallelizes the quantum algorithm and thus uses more qubits, but each qubit requires a lower quality than before. This is due to the fact that fewer operations are performed on the individual qubits and the structure of the algorithm is much more robust to certain errors in the system. With the new approach, we only need a success rate of around 99.99% per operation. This is of course much lower than the previously required 99.999999% success rate.
So in summary, quantum computing still has a few stumbling blocks to overcome before it can be used commercially for the applications described above. However, the latest developments from JoS QUANTUM give hope that quantum computing will have more and more applications in a wide variety of cases, even with systems that cannot completely combat their errors.
References
M. C. Braun, T. Decker, N. Hegemann, S. F. Kerstan, and C. Schäfer, “A quantum algorithm for the sensitivity analysis of business risks,” arXiv:2103.05475 [quant-ph], 2021.
E.Pelofske, A. Bärtschi, S. Eidenbenz, “Quantum Volume in Practice: What Users Can Expect from NISQ Devices, “, arXiv:2203.03816v3 [quant-ph], 2022], https://arxiv.org/pdf/2203.03816.pdf
M. C. Braun, T. Decker, N. Hegemann, S. F. Kerstan, “Error Resilient Quantum Amplitude Estimation from Parallel Quantum Phase Estimation”, arXiv:2204.01337v1 [quant-ph], 2022, https://arxiv.org/pdf/2204.01337.pdf
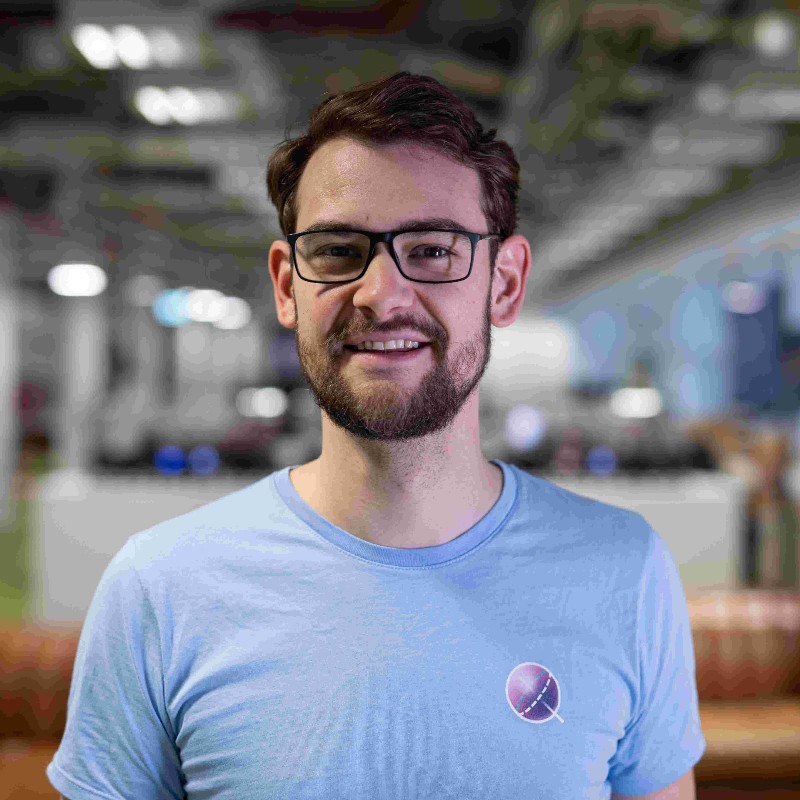
About the author
Niklas Hegemann is managing director of JoS QUANTUM. Before he worked as a management consultant in different business and technical areas focusing on software integration and financial services. He was responsible for several projects mostly in the field of trading and risk management. Niklas worked at the German electron-synchrotron DESY where he used Monte Carlo simulation methods to validate high energy experiments. He received his degree (Dipl.-Phys.) with subsidiary subject economics in 2011 from University of Hamburg.